Introduction
Since the explosion of Generative AI (GenAI) in 2022, daily breakthroughs in AI features and solutions have become the norm. Keeping pace with this change is a formidable challenge. This shift mirrors the space race of the 20th century, with GenAI emerging as a modern Sputnik, poised to redefine entire industries.
In this high-stakes race, tech companies are moving swiftly to deploy advanced AI models capable of autonomous reasoning and action. At the edge of this revolution is Agentic AI — autonomous agents designed to handle repetitive work with limited human oversight.
Evolution of GenAI in Content Management: From Assistants to Agents
In the three years since GenAI’s rapid integration into content operations, there have already been two distinct waves of AI transformation.
First Wave (2022–2024)
This initial phase saw the widespread adoption of Generative AI (GenAI) solutions designed to assist us in content-related tasks, as well as integration of Gen AI features into other content operations tools such as content authoring tools and automating review tools. These assistants and AI-infused operations tools support content creation, editing, version control, review and approval leading to the generation of derivative materials at a faster pace. Although still reliant on human input and oversight, the assistants and tools represented a step forward in streamlining the content development process.
Second Wave (2025+)
Building on the foundations laid by Gen AI tools, the current wave is characterized by the rise of AI agents—autonomous systems capable of executing complex content workflows with minimal human intervention. These agents can independently manage end-to-end processes such as content assembly, regulatory alignment, version control, and multi-channel distribution, with minimal human involvement. Agentic AI marks a shift from assistance to autonomy, relieving us of repetitive tasks and to allow us to upskill and focus on more strategic work.
What differentiates the two waves are: support versus autonomy. Whereas the Gen AI assistants and tools help to reduce production time, it always plays a supportive role. Agentic AI solutions on the other hand are more autonomous due to a smart system referred to as the ‘brain’. It enables the agents to reason, plan and retrieve both static and dynamic data with minimal human intervention.
Deploying an agentic system offers many business benefits to organizations. One of the most significant is the ability to modernize content infrastructure without requiring a complete system overhaul. “Agents will essentially become the middleware connecting all content systems,” explains Jason Sundberg, Commercial Content Strategist, and a member of The Stem network.
Assistants versus AI Agents
The assistants and AI agents both run on a generative AI foundation. However, the AI agent provides greater independence, flexibility and adaptability compared to the assistants.
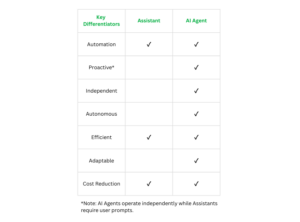
When to Pilot AI Agents
The first step should be identifying a high-need, high-impact project to conduct a pilot. This could involve creating a more seamless method for repurposing content across different channels and communication objectives, managing version control, or ensuring a submission-ready document for MLR (Medical, Legal, and Regulatory) review. Regardless of the approach, the benefits are clear: reduced time to market and significant cost savings.
Joe Falcone, Content Operations Excellence Consultant at The Stem, sees the value in piloting these solutions sooner rather than later. “At first, these Agentic AI solutions are going to be pretty basic, just as we saw with the GenAI solutions and tools. But we also saw how fast these solutions improved over time. We’ll see which solutions survive the shakeout, but it’s worth doing a pilot to get the solution integrated into the organization.” And there is an appetite for doing pilots according to Joe because “…some teams that are thinking of creating modular content hubs are thinking of skipping modular entirely and going all-in on AI. While that sounds nice, the reality is that we should be doing both as they are complimentary not in competition, and getting these pilots off the ground sooner rather than later will likely yield rewards.”
Possible scenarios include:
A brand approaching Loss of Exclusivity (LOE) requires aggressive marketing and sales efforts but does not need new label or messaging updates. AI-driven content repurposing can maximize the impact of existing assets while optimizing distribution across channels.
A small brand with a limited marketing budget must generate omnichannel content efficiently and cost-effectively. AI-powered automation enables scalable content creation while maintaining consistency and compliance.
A regulatory label update that does not alter the brand concept may require slight messaging variations to reflect the change. AI-assisted content adaptation ensures accuracy, speeds up the update process, and streamlines MLR review.
A global brand with local adaptation must maintain a singular branded concept while ensuring regionally relevant messaging and compliance with local regulatory guidelines. AI can help tailor content for different markets without compromising brand integrity.
Sales enablement efforts require personalized digital content, including emails and Interactive Visual Aids (IVAs), tailored for specific healthcare professionals. AI-driven customization enhances engagement and ensures alignment with sales strategies.
Rare disease brands with limited budgets face challenges in patient identification and disease education. AI-powered content systems can help create targeted, educational materials to support awareness, diagnosis, and engagement efforts efficiently.
Patient access programs with regional differences by state require precise, localized content to help patients navigate insurance coverage, copay assistance, and reimbursement pathways. AI can generate region-specific materials that account for variations in state policies, payer requirements, and patient assistance programs, ensuring accurate and up-to-date information for patients and healthcare providers.
Illustrative Example of Agentic AI Derivative Content Development Workflow
To provide an example, the image below shows how an Agentic system could streamline the development of derivative content using 7 specialized agents, each responsible for a specific task within the content creation pipeline. Once deployed, these agents, working together, complete the entire end-to-end workflow up to MLR submission, at which point a human gets involved. For a detailed explanation of what the agent is actually doing, refer to the end notes.
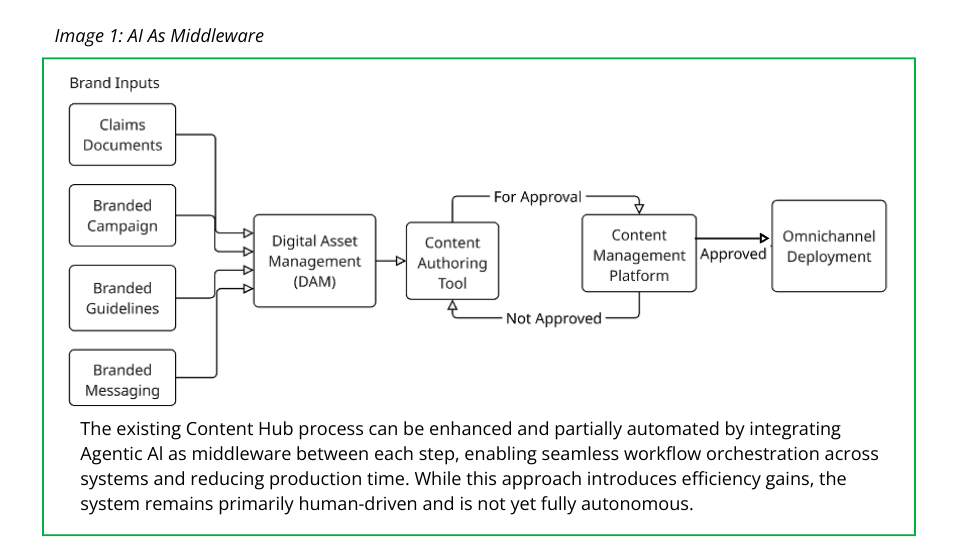
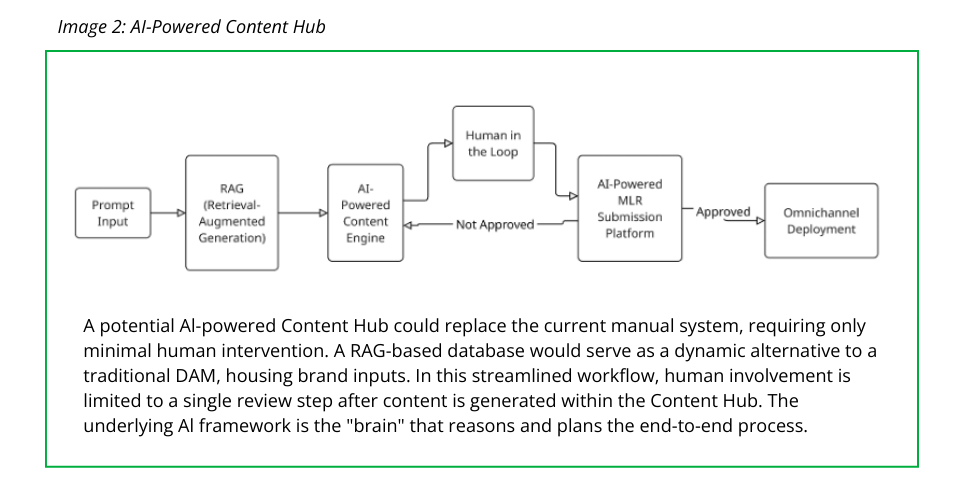
The benefits of this agentic system are speed of completion: From the initial input to the first human intervention, the system will require up to 5 minutes, instead of hours or days.
While the workflow is largely automated to minimize human involvement, human input remains essential at key points—particularly at the beginning and end of the process, as the content is finalized for MLR review.
Who Should Oversee Agentic Systems
Depending on the content maturity of Biopharma and Life Sciences companies, content operations are a shared responsibility including content operations, IT and omnichannel teams. Similarly, the decision to deploy and responsibility for setting up agents should be taken by this cross functional team. Once active, a decision needs to be made as to who would manage the agents. For a larger company, this could fall to the content factory function or marketing operations or for a smaller company agentic management could become a junior brand marketer responsibility. Note also that agents have the impact of reducing dependence on expensive human resources involved with content factory contracts.
How to Get Started
Introducing Agentic AI into content operations doesn’t require a full-scale overhaul. There are data challenges that need consideration with respect to data, metadata and business rules. It starts with a clear understanding of where these solutions can deliver the most value—and building from there. Below are key steps to guide the initial adoption:
- Identify a high-impact use case
Start with a workflow that is both resource-intensive and ripe for automation. This could include content repurposing, version control, label updates, or preparation for MLR review. These areas offer clear opportunities for efficiency gains, faster execution, and measurable outcomes.
- Evaluate existing infrastructure
Take stock of current systems, workflows, and team responsibilities. Understanding your content ecosystem will highlight where integration with Agentic AI can streamline operations without creating disruption.
- Align cross-functional stakeholders
Content operations often sit at the intersection of regulatory, brand, medical, and IT. Early alignment with these groups ensures shared goals, proper oversight, and smoother implementation. Collaboration from the start accelerates adoption and increases the likelihood of long-term success.
- Define success criteria
Establish what success looks like—whether that’s reduced time to market, improved compliance accuracy, or cost savings. Clear metrics help evaluate the performance of AI systems and guide future scaling decisions.
- Conduct a focused pilot
Test the system on a defined use case with manageable scope. A pilot provides a low-risk environment to assess real-world application, integration with existing tools, and the agent’s ability to operate with limited human intervention.
- Learn, refine, and scale
Use insights from the pilot to fine-tune processes, identify additional opportunities, and gradually expand the agent’s role across content workflows. This stepwise approach ensures each phase builds on real outcomes, not assumptions.
- Build team readiness
As Agentic AI assumes more of the executional workload, content teams will shift toward strategic oversight and orchestration. Preparing teams for this change through training and upskilling will unlock new value and drive adoption.
- Leverage specialized consulting partner for project strategy and set-up
Working with a consulting firm that understands both the AI landscape and the regulatory demands of life sciences can accelerate implementation. These partners offer strategic insight, technical know-how, and real-world experience to guide AI integration effectively.
Conclusion
Agentic AI marks a new era—not just for content workflows, but for how life sciences organizations operate at scale. Unlike earlier GenAI tools that required continuous human input, Agentic AI introduces autonomy, adaptability, and intelligent orchestration into processes that were once manual, repetitive, and siloed.
This isn’t about adding yet another tool to the tech stack—it’s about introducing the connective tissue to improve how work gets done. From accelerating time-to-market to enhancing regulatory precision, Agentic systems have the potential to fundamentally transform operational efficiency across the enterprise.
For companies ready to lead, the time to pilot is now. Early adopters will not only shape the standards of AI integration in life sciences—they’ll gain a competitive edge that compounds over time.
Appendix:
Initial Input: The workflow begins with “User Instructions” (blue box on left), which serves as the starting point for content creation.
Data Processing: Instructions are processed through an agentic workflow that sends the instruction to a RAG Retrieval System
RAG Retrieval System: A Retrieval-Augmented Generation system accesses relevant information (i.e. text, images, ISI boilerplate, indication, claims document etc.)
Human In the Loop: The human in the loop allows a team of stakeholders representing various disciplines to review and provide comments on the generated content. Following the review, the comments are uploaded into the AI process and automated revision occurs. At this point, the AI is instructed on the format type of each of the assets and dimensions of the content.
MLR Decision: The final decision diamond appears to be “MLR Approval” (Medical, Legal, Regulatory).
Completion: The workflow concludes with Launch Materials and omnichannel deployment of content.
Authors
Gregg Fisher, Managing Partner, The Stem – gfisher@thestem.com
Noriko Yokoi is a Lead Strategy Consultant, The Stem